The Role of Machine Learning in Modern Data Science
Data science is a domain that studies several sources of data and employs a range of techniques, tools, systems, and algorithms to understand both structured and unstructured data through observation. That understanding is then utilized by businesses, the government, and other such entities to enhance profits, innovate new products and services, enhance public service processes, and so on.
Data science is a field that combines several things to mine data for its usefulness, including statistics, data analysis, scientific methods, artificial intelligence, and many other fields. Knowledge applied in different ways enables data scientists and data engineers to sift through and formulate data from diverse external and internal systems, such as the internet, consumer and mobile devices, and so forth.
Importance of Data Science
In the present context, data facilitates effective decision-making and calls for analysis. Data science is used to deal with large volumes of raw data to recognize diverse and often latent trends, derive insights and inform business decisions.
The following elucidates the importance of data science:
1. Revealing valued and unfamiliar trends
Do you want free career counseling?
Ignite Your Ambitions- Seize the Opportunity for a Free Career Counseling Session.
- 30+ Years in Education
- 250+ Faculties
- 30K+ Alumni Network
- 10th in World Ranking
- 1000+ Celebrity
- 120+ Countries Students Enrolled
Data science is capable of breaking the barriers and drawing more relationships and patterns within data. In this case, new perspectives may be drawn from this knowledge. Such knowledge will then enable us to make sound conclusions and decisions.
2. Aids in the creation of new products and benefits
As we have noted, problems and concerns hidden or overlooked in many instances can be revealed with data analytics. Enriching knowledge of departments or roles, customer purchasing behavior or feedback, and even company practices can bring about creativity in both internal and external solutions.
3. Prediction and optimization at a glance
In addition, the elements of data science can also be incorporated within the time frame. The objective of this is achieved across the board in all fields.
What is Machine Learning (ML)?
One of the branches under the domain of Artificial Intelligence is Machine Learning which allows computer programs the ability to use basic reasoning to learn from data over time and make forecasts without much help from humans. Numerous techniques are incorporated into machine learning in the course of which these algorithms seek insights in large volumes of data and make useful information out of them.
Data Science vs. Machine Learning
DATA SCIENCE | MACHINE LEARNING |
Data science is all about processes and systems to extract data from structured and semi-structured data. | Machine learning (ML) is a field of artificial intelligence (AI) that allows software to learn from data to identify patterns and make predictions automatically with minimal human intervention. |
Data science deals with data. | Machine learning utilizes past experiences to learn about the data. |
Data gathering, manipulation, data cleaning, etc. are data science operations. | ML is of three types: supervised learning, unsupervised learning, and reinforcement learning. |
Example: Netflix using data science is an example of this technology. With the help of Data Science, Netflix can provide users with personalized recommendations on movies and shows. It can also predict the original content’s popularity with trailers and thumbnail images. | Example: Facebook using ML is an example of this technology. With the help of machine learning, Facebook can produce the estimated action rate and the ad quality score, which is used for the total equation. ML features such as facial recognition, targeted advertising, language translation, and news ed are also used in many real-case scenarios. |
Why is Machine Learning Important?
Data is worthless unless it is transformed into information that can be used. Both businesses and customers have data that can be efficiently managed with machine learning to support decision-making, giving the organization a greater competitive edge.
Therefore, it compromises predictive modeling and machine learning, which are crucial for data science operations. Data scientists need to understand the concepts and principles of machine learning to make precise estimations and predictions.
Do you want free career counseling?
Ignite Your Ambitions- Seize the Opportunity for a Free Career Counseling Session.The techniques can be employed in curbing expenses, minimizing dangers, and enhancing one’s lifestyle, among other uses, such as recommendations, cybersecurity incursion prevention, autonomous vehicles, and many others. That’s where once again, machine learning is needed.
What does machine learning contribute to Data Science?
Data Science as a discipline is about reading from raw data as much as possible. This entails disintegrating the data and reshuffling it in such a way that the relations among the obtained blocks can be assessed. Algorithmic approaches explore that data and process it so that the final output is the information that can be used by the company to solve a particular problem.
When it comes to Data Science, the paradigm of Machine Learning is introduced at the stage when we want to determine something accurately concerning the information at hand, e.g., deciding if a person is likely to have cancer.
Here are the 9 steps that take place within machine learning for data science.
1. Understanding Business Problems
To establish a working business model for the client, the best bet will be to first ascertain what business problem the client is facing. Let us assume in this case that the client wishes to conduct a diagnosis of whether a patient is likely to have cancer or not. In such a scenario, domain experts understand the underlying problems that are present in the system.
2. Data Collection
After understanding the problem statement, you have to collect relevant data. Depending on the business relation, machine learning is efficient in sourcing and processing structured, unstructured, and semi-structured data from various databases on different systems.
3. Data Preparation
The first stage of the data preparation process is data cleaning. It is a very crucial step in data preparation. In data preparation, duplicates and nulls, inconsistencies, invalid, incomplete, and badly formatted data are cleaned up and removed.
4. Exploratory Data Analysis (EDA)
Exploratory Data Analysis helps to find meaningful information that will be beneficial at the next step of the Data Science life cycle. The reason why EDA is imperative is because EDA is capable of finding outliers, anomalies, and even trends in a given data set. Such understanding may assist in establishing how many features are sufficient to create a model.
5. Feature Engineering
Feature engineering is one of the important steps in a Data Science Project. It helps in creating new features and transforming and scaling the features. In this area, Imagination is very helpful in drawing from what has been found in the data exploration step.
6. Model Training
In this model training, we fit in the training data, this is the part where “learning” begins. The model is trained on training data and the testing system performance is on system testing data which is also known as unseen data.
7. Model Evaluation
After completion of Model Training, the next step is to test the Model. This means that testing your Model with a different dataset provides insight into the performance of your Model with future data.
8. Hyperparameter Tuning
Once a Model is claimed to have been trained and assessed, the Improvement of the Model’s Performance could also be done by changing some of the hyperparameter settings. It is important to conduct a hyperparameter tuning of the model to achieve the intended goal of the model.
9. Making Predictions and Ready to be Deployed
This is the last stage of any machine-learning task. This is where the machine responds to every one of your questions by its acquired learning. Following a series of precise forecasts, the Data Model is primed for use.
Future of Data Science
The profession of a data scientist entails dealing with large volumes of information to analyze and address different issues. It is nearly impossible to find a field that operates in the absence of data science, be it business, healthcare, education, or even advertising. In this case, the importance of data quality can never be emphasized enough since it helps ensure the relevance of machine learning. Due to the increase in the digital space and data being generated at a very high rate, Data Science is in high demand. Machine Learning is still and will remain a critical component in the field of Data Science. In practical terms, this artificial intelligence technology will make it possible for companies to process massive datasets to seek and identify relevant factors that will inform decision-making.
Conclusion
Data is important for us. We all know data science is becoming more important day by day, and with this, we must also consider that the value of data is also increasing.
Data science and machine learning have notable applications in day-to-day activities as in cases of any online recommendation systems, speech recognition applications (like Siri and Google Assistant), as well as any online transactions where it is possible to detect fraud. Therefore, Data Science and Machine Learning will to become among the most important technologies in the modern world.
In simple terms, there will be an upsurge in Data Science job vacancies in all parts of the world. That is, it is the most appropriate time to make up one’s mind and venture into the field and acquire relevant and valued knowledge. If you want to learn Data Science through practice and increase your chances of landing an excellent-paying Data Science job, then you should sign up for AAFT School’s Data Science Course!
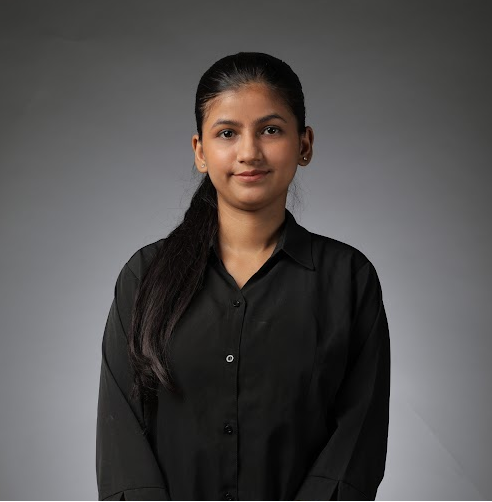
Deepika Joshi is a dedicated content writer and specialist with over three years of experience in the education and academic niche. Currently pursuing her master’s degree in Advertising and Public Relations, she also holds a Post Graduate Diploma in Public Relations and Events. Deepika excels at creating engaging, informative, and persuasive content that resonates with diverse audiences. She is well-equipped to tackle new challenges in the dynamic world of growing content.