Top Data Science Tools You Need to Know in 2025
As 2025 knocks on the doors, several Data Science tools and platforms are set to become industry leaders in transforming how data is leveraged by modern businesses for decision-making.
Whether you are an experienced professional who is looking to upgrade your skills or a beginner eager to explore this dynamic field, now is the golden ticket for you to enroll in a Data Science course like B.Sc in Data Science.
In this blog, we will explore some of the top and happening Data Science tools to watch out for in the year 2025 that will shape the future of Data Science. Letās kickin ‘our journey!
Top Trending Data Science Tools in 2025
Several data science tools impress you through their incredible applications. These tools will be revolutionizing the data analytics and decision-making industry. Here are the top tools and platforms to watch out for in 2025:
A) Programming Languages
Do you want free career counseling?
Ignite Your Ambitions- Seize the Opportunity for a Free Career Counseling Session.
- 30+ Years in Education
- 250+ Faculties
- 30K+ Alumni Network
- 10th in World Ranking
- 1000+ Celebrity
- 120+ Countries Students Enrolled
Programming languages are the most important Data Science tools because they provide the means to perform various data analysis tasks. These languages come with their own sets of libraries and frameworks that work to simplify complex computations, manipulation of data, and visualization. Such features make them an incredibly versatile prospect for Data Scientists worldwide.
1. Python
It wonāt be wrong to say that Python is the most popular and widely used Data Science programming language of the 21st century. The best part about this tool is its user-friendliness and readability, which resembles a common English language. This feature makes it the top choice for a variety of Data Science tasks, such as data manipulation and data visualization.
Python can also perform various other levels of data-related tasks, such as data manipulation and analysis with libraries such as Pandas and NumPy, and for implementing algorithms related to Machine Learning (ML).
Book Now ā
2. R Language
R Language is another extremely useful programming tool for data scientists. It is known for its proficiency in statistics and ggplot2 data visualization. Further, it comes with a vast collection of libraries and packages, especially for performing complex Exploratory Data Analysis (EDA) and advanced statistical modeling.
Read Also: How will AI & Machine Learning Redefine Data Science in 2025?
R Language is typically used for statistical analysis as well along with data cleaning and transformation with dplyr and tidyr libraries, and building statistical models to effortlessly perform Hypothesis Testing and Regression Analysis.
Do you want free career counseling?
Ignite Your Ambitions- Seize the Opportunity for a Free Career Counseling Session.3. MATLAB
Though it’s not the core specialized for data scientists, yet the knowledge about MATLAB can take you a long way forward in your Data Science journey. It is a Programming language that is specifically designed for performing numerical data analysis and computing. It has built-in functions and toolboxes that are made for various high-level and complex Data Science tasks.
Moreover, MATLAB can easily create visually compelling data visualizations and build Machine Learning (ML) algorithms for Regression Analysis, classification, and clustering.
b) Data Query and Management Tools
Data query and management tools are crucial for handling and manipulating large amounts of data (Big Data) efficiently from massive databases. These tools enable data scientists after completing their Data Science Bachelor’s degree Course to perform data extraction, cleaning, and data organization from various sources, making sure it is always ready for performing data analysis.
1. SQL
Structured Query Language (SQL), is an important data management and database manipulation tool. It is used to perform data extraction, transformation, and various types of other analysis within relational databases.
Moreover, SQL can retrieve Relational Database data easily, perform data cleaning and integration datasets from various sources, and facilitate data exploration to identify any patterns and anomalies.
c) Data Visualization Tools
Data visualization tools help to transform raw data into visual formats, making it seamless to understand and communicate complex insights to the users. These tools are essential for displaying interactive dashboards and report creation for seamless and effective data-driven decision-making.
1. Tableau
Tableau is a data visualization tool for Data Science professionals, after completing their B.Sc in Data Science to create engaging dashboards and reports. It also enhances their process of data exploration and enables the simplification of complex insights communication to stakeholders.
Read Also: How to Develop a Data-Driven Mindset: Tips for Aspiring Data Scientists
The main usage of Tableau is to enable easy dashboard creation for complex datasets, data visualization, and performing efficient real-time analytics through live data connection.
2. PowerBI
PowerBI will continue to lead as a powerful tool for data visualization. It is expected to remain a top choice for raw data into meaningful insights transformation through interactive dashboards and reports.
Among the key features of PowerBI include real-time data visualization, extensive data connectors support, and Microsoft Office Suite integration.
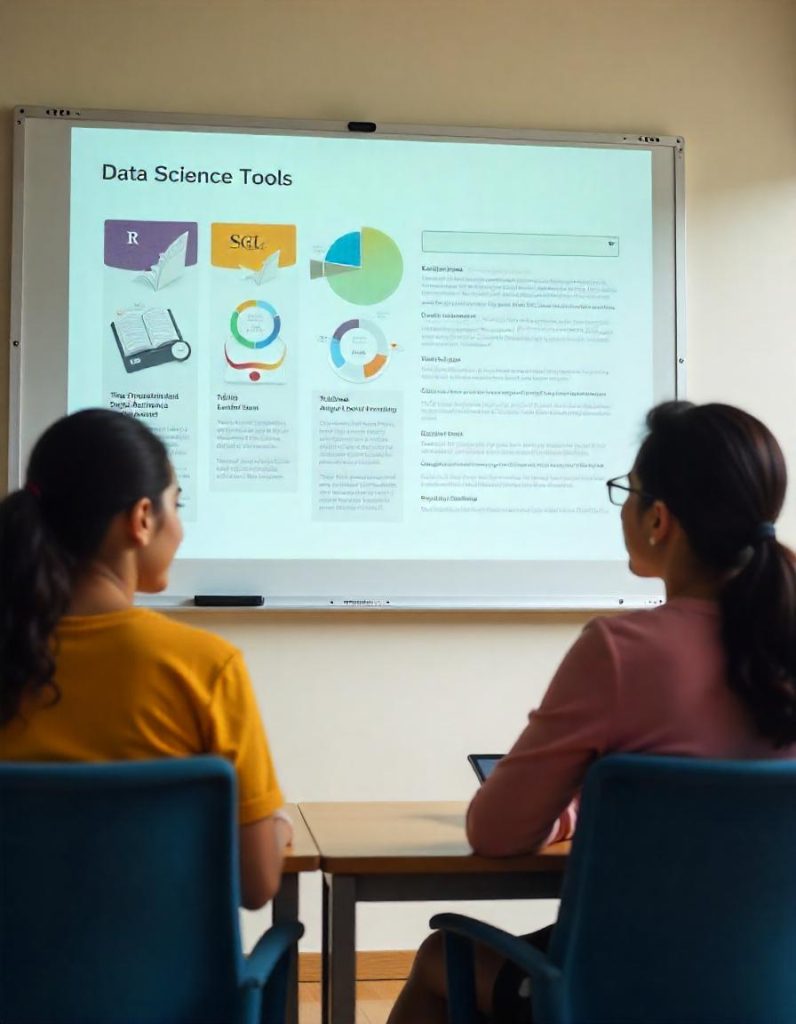
Read Also: The Role of Machine Learning in Modern Data Science
d) Machine Learning (ML) and AI Tools
Machine Learning (ML) and AI tools are pivotal for developing predictive models and data analysis algorithms. It can easily recognize patterns and make informed predictions. These tools also support a wide range of applications, which include Natural Language Processing (NLP) and image recognition.
1. TensorFlow
TensorFlow was developed by Google and is an important open-source platform that assists Data Science professionals after completing their Bachelor of Data Science in computational representation as graphs. It provides the flexibility and efficiency needed to train and build Neural Networks and other Machine Learning (ML) models.
TensorFlow is used for recognizing images and computer vision, performing Natural Language Processing (NLP) tasks like text classification and sentiment analysis, and Time Series Analysis for randomized data such as stock prices and weather patterns.
2. Microsoft Azure Machine Learning (ML)
As there is significant growth in cloud computing, Microsoft Azure Machine Learning (ML) will remain a top platform for building, deploying, and managing models for machine learning (ML). It provides a fully integrated environment with seamless Cloud-based services. These include automated Machine Learning (ML) (AutoML) and scalable Cloud infrastructure.
3. H2O.ai
H2O.ai will remain a leading AI and Machine Learning (ML) development platform. With its AutoML and cloud-based AI services, it will continue to stay relevant to data scientists. These features enable them to build predictive models without requiring high-level coding proficiency.
Read Also: Data Science Courses After 12th Fees, Duration & Eligibility
Some of the other key features of H20.ai include AutoML, seamless Hadoop and Spark integration, and robust data visualization tools.
4. Databricks
Databricks is built on Apache Spark and leverages big data and AI to perform data simplification and streamline the Machine Learning (ML) lifecycle. This key tool is predicted to be a key player in 2025, especially for those who are unifying their analytics and AI efforts together.
Some of the critical features of DataBricks include a unified analytics platform, Machine Learning (ML) workflow optimization, and collaboration in real-time.
5. KNIME
Konstanz Information Miner (abv KNIME) is expected to remain as a preferred open-source platform for creating data pipelines, analytics workflows, and Machine Learning (ML) models. Furthermore, its simplicity and wide extension ranges make it accessible for data scientists at all levels.
Some of the robust features of KNIME include drag-and-drop workflow creation and integration with popular Data Science tools like Python and R.
6. RapidMiner
RapidMiner will remain popular for end-to-end Data Science workflows. It provides an intuitive platform for creating Machine Learning (ML) models. This makes it accessible to both Data Scientists and Business Analysts.
Read Also: How Data Science is Transforming in Every Field?
Some of the profound and widely acclaimed features of RapidMiner include the preparation of drag-and-drop data and integration with popular data visualization platforms.
Conclusion
In 2025, these top Data Science tools and platforms will remain pivotal to engraving the future of data analytics, AI, and Machine Learning (ML). Therefore, it’s important to stay thoroughly updated with these tools. This will not only help you remain industry-competitive but also unravel new innovation and growth opportunities.
For more information on Data Science tools or if you are looking to enroll in any of our Data Science Courses like B.Sc in Data Science, connect with our AAFT team today!
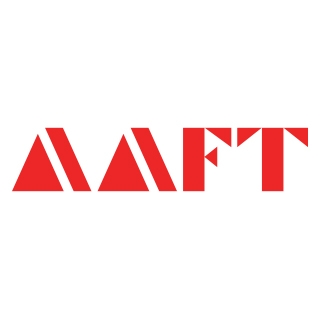
AAFT has been providing the world with limitless creativity and expression since 1993! Through a dynamic and industry-driven curriculum, AAFT provides engaging and captivating articles to persuasive blogs and empowers its readers to explore diverse avenues of creative media education-related content.